TL;DR
Since the release of OpenAI o1, Test-time Computing has become a hot topic.
While I'm still exploring my research direction for the second year, I want to focus on understanding this technology for a while.
On the personal side, I enjoyed the Halloween season (photo is a group picture taken at the lab on Halloween).
1. Research
Scaling Laws of LLM (Training)
It’s well known that the discovery of scaling laws for training-time computing has justified and sustained current AI development investments.
- 1*: The classic concept of overfitting in traditional machine learning doesn’t necessarily apply to neural networks or LLMs, where parameters are identified via probabilistic learning rules. The fact that the performance improvement degree is predictable is also surprising.
- 2*: This discovery has also driven innovations in LLM training pipelines, allowing meta-parameters to be optimized with a smaller LLM and then used to train a larger LLM only once, based on confirmed scaling laws.
- 3*: The scaling laws underlie NVIDIA's massive GPU purchases, driving the company's valuation to the highest in the world.
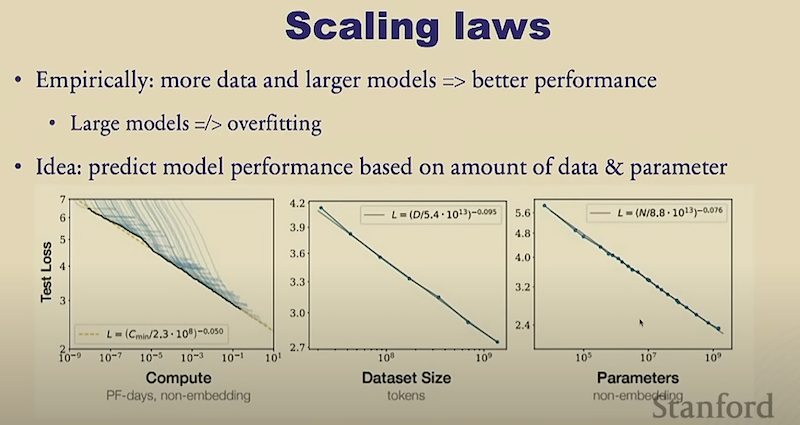
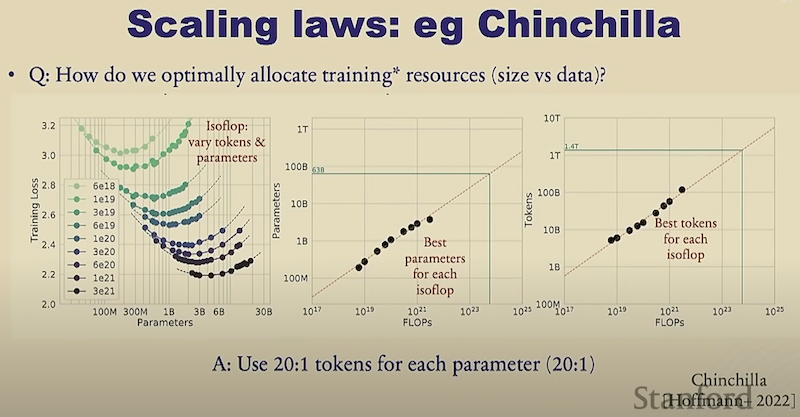
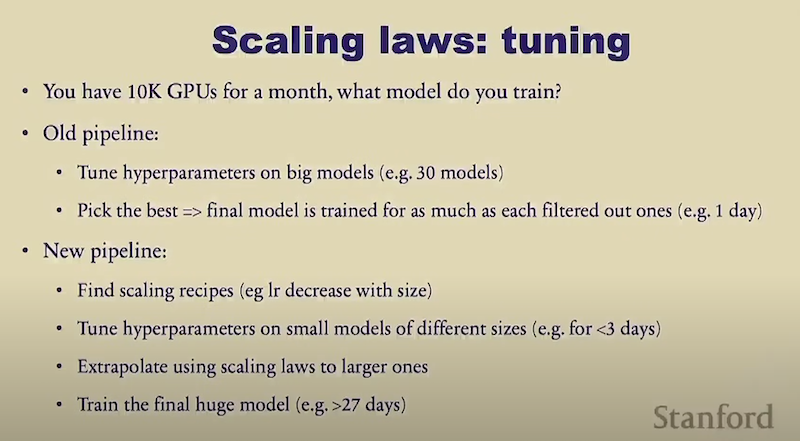
Test-time Computing
Currently the hottest AI research topic.
Karpathy’s YouTube explanation on the Deepthinking Unit and the Dual Process Theory from neuroscience suggests that LLMs predicting only the next token aren’t enough. OpenAI’s o1 release proves this.
The larger impact lies in demonstrating that scaling laws also apply to inference computing. However, since the algorithm remains undisclosed, many are eager to uncover the secret behind it.
In our lab, a Reading Group on papers related to Test-time Computing has been launched.
Research Direction
I am exploring my future research direction.
Key data in the manufacturing sector are time-series data and image data obtained from IoT.
In the first year, I focused on image data x Data-Centric AI x GenAI.
I released VIHub, an MLOps service for image AI development.
Developed features applying Data-Centric AI concepts, including Digital Spec Catalog, Miss-Label Detection, Duplication Detection, Semantic Image Search,
and Spec Document Verification combined with Multimodal LLM.
While I haven’t decided on my direction for the second year, I want to pursue "Test-time Computing" for now.
- Lamma 3.2 release (first Multimodal-supported base model in open source) encourages exploring its practical application to edge image AI.
- Continuing development of VIHub is also a candidate.
- Additionally, it could be worthwhile to explore GNN x time-series data.
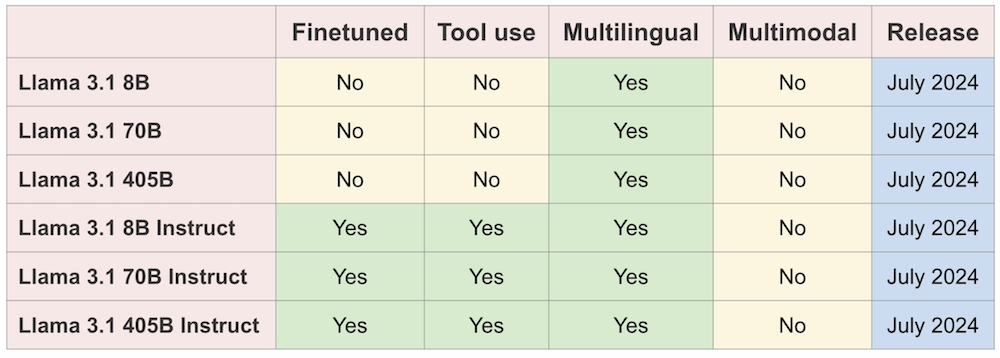
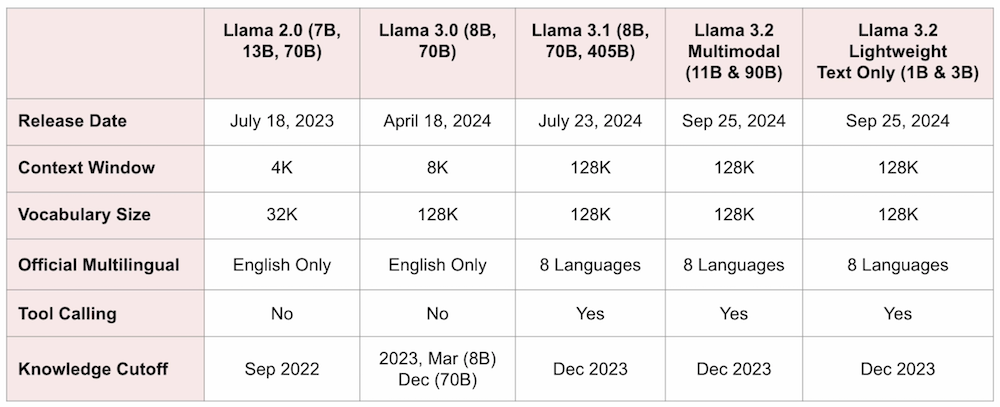
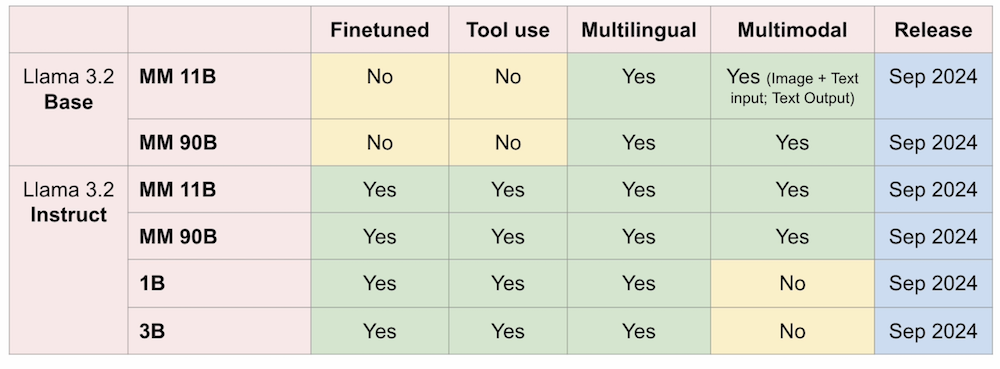
Applications of Test-time Computing
Though Test-time Computing is time-consuming at inference, it has a broad range of applications if real-time performance is not required.
With increased AI performance, emergent phenomena allow more tasks to be entrusted to AI.
Test-time Computing is particularly effective for problems that require “deep thinking,” such as coding automation.
It could also involve giving log data to an AI to build a mathematical model and perform causal inference.
The development of such platforms or SaaS solutions is worth considering.
In theoretical science research, having AI think deeply about models explaining experimental data might lead AI to make discoveries in fields like theoretical physics, where the human role may become focused on verification.
2. Life
10/17 🇯🇵 Election @ SF Embassy
10/20 Farewell Party for 🇨🇳 Lucas
10/27 Diwali Party
10/31 Health Check @ 🇺🇸 Hospital
The new semester has started, and I’ve joined some ESL classes.
I got to experience voting in the Japanese House of Representatives election and undergoing a health checkup in the U.S.
I had the unique opportunity to observe an American election on-site, which I’ll detail next month.
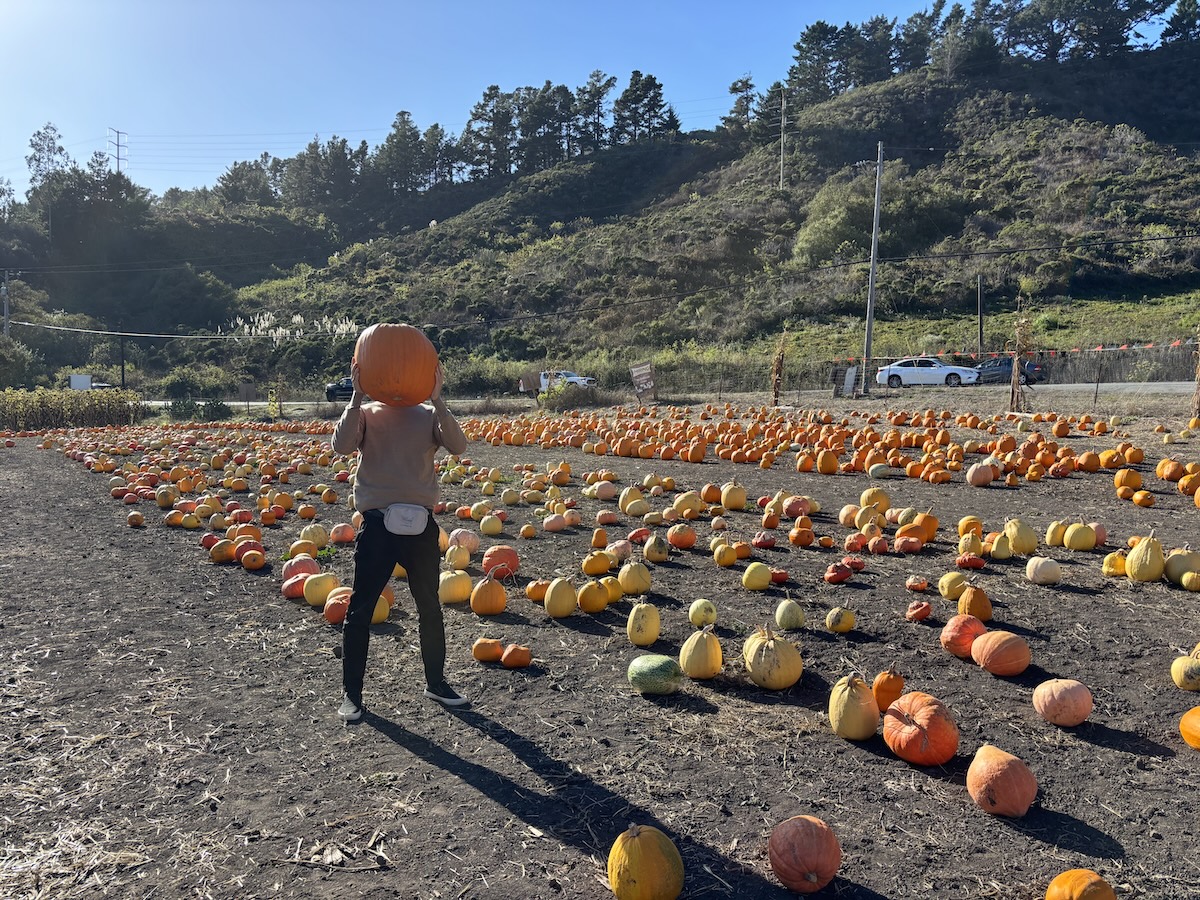
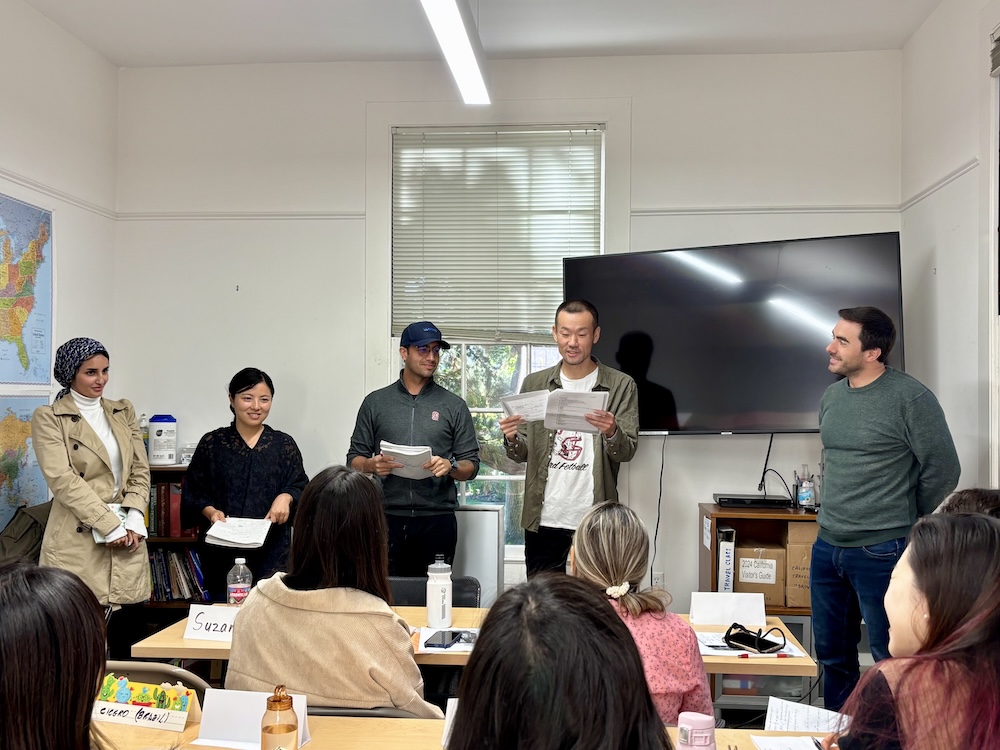
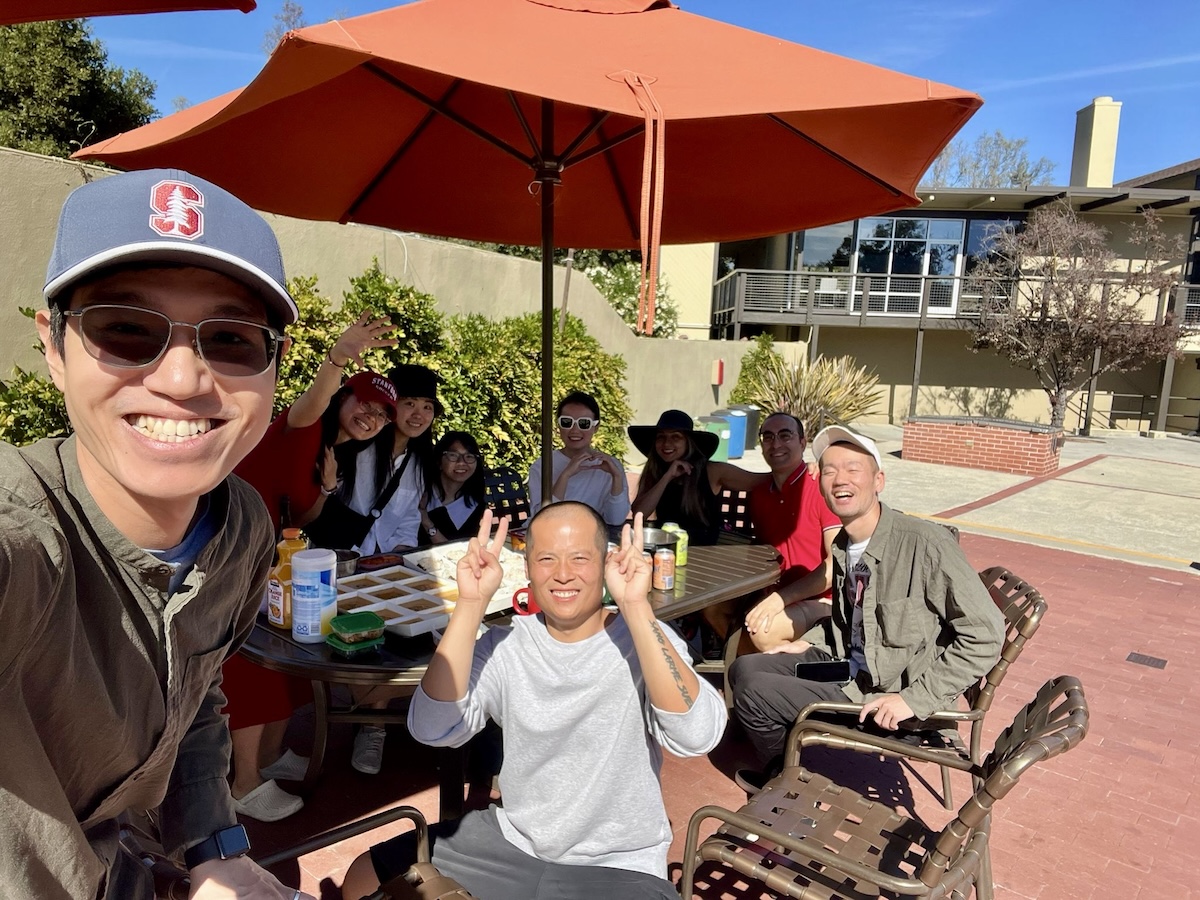
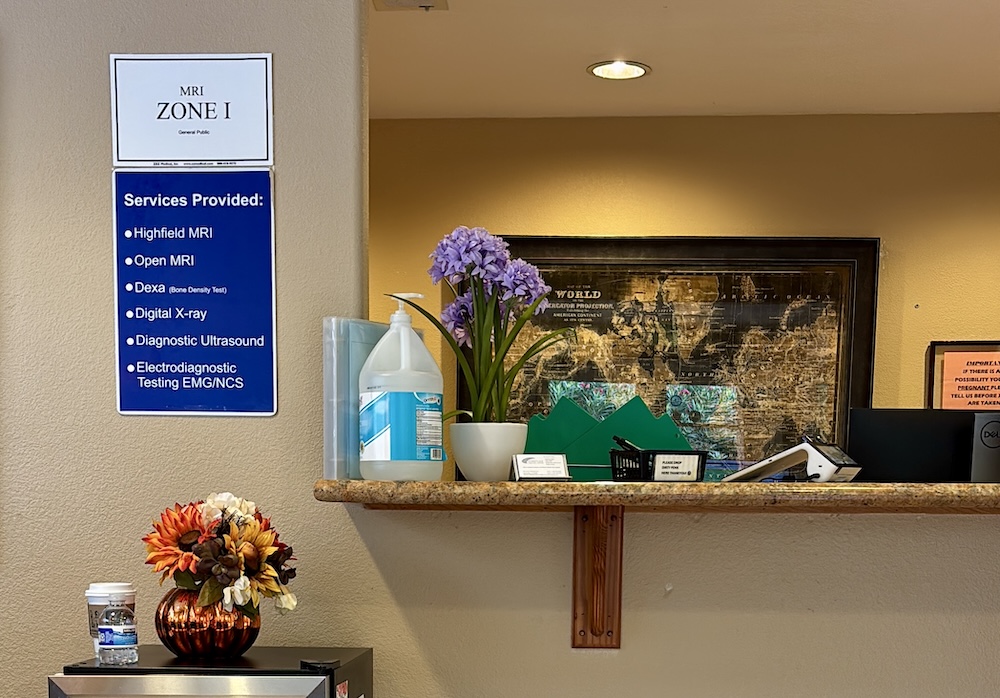